1 Matrix operations
There are three basic matrix operations that would be part of any GPU
matrix toolkit:
1. The inner product of two vectors c = a · b.
2. Matrix-vector operations: y = Ax.
3. Matrix-Matrix operations: C = A + B, D = AB, E = A−1.
A number of problems can be solved once one has these basic operations
(especially in physical simulations). This is one of the most studied problems
on the GPU.
2 The Inner Product
Consider the inner product c = a · b, which we rewrite as
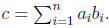
2.1 Technique 1: Small memory
Each vector is stored in a 1D texture. In the ith rendering pass, we render a
single point at coordinates (0,0), having a single texture coordinate i . The
fragment program uses i to index into the two textures and returns the value
, where s is the running sum maintained over the previous i − 1
passes. Note that since we cannot read and write the
location where s is
stored in a single pass, we use a ping-pong trick to maintain s.
This procedure takes n passes, and requires only a fixed number of texture
locations (excluding the storage for a and b).
2.2 Technique 2: Fewer passes
The second technique uses more working memory (n units), but requires
fewer passes. We write a and b as 2D textures (2D textures allow for more
storage, since the dimension of a texture is typically bounded, and are better
optimized by the rasterizer).
We now multiply the contents of the textures, storing the result in a
third texture c. This can be done with a simple fragment program that takes
the fragment coordinates and looks up the a and b textures, returning their
product. We render a single quad in order to activate the fragment program.
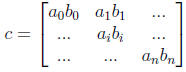
Finally, all the numbers in c must be summed together .
This can be done
in log n passes , using a standard reduce operation.
This procedure takes only log n passes , but requires 3n units of texture
memory.
3 Matrix-Matrix operations
We can store matrices as 2D textures. Addition is trivial .
3.1 Multiplication
3.2 Technique 1: The Basic Approach
”Fast matrix multiplies using graphics hardware ” by Larsen and
McAllister”
Express multiplication of two matrices as dot product of vectors of matrix
rows and columns. That is to compute some cell cij of matrix C, we take the
dot product of row i of matrix A with column j of matrix B:
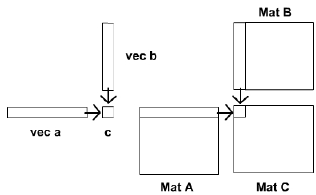
1st program used multitexturing and blending, each plane
would compute
each place in the answer. In 1st pass: AB :
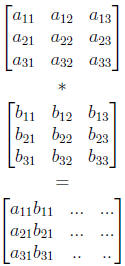
We can use inner quad idea to do this:
pass 1
if at location (x, y)
output 
pass 2
output 
pass k
output 

1)uses n passes
2)space N = n2
3.3 Technique 2: A Speedup
”Dense Matrix Multiplication” by 
To make it faster:
Instead of making one computation per pass, compute multiple additions per
pass in fragment program:
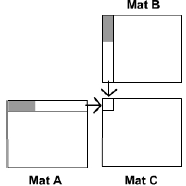
Pass 1 becomes: output 
Must consider that there is a tradeoff between the length of fragment
program vs. the number of passes.
3.4 Technique 3: Using All Channels
”Cache and Bandwidth Aware Matrix Multiplication on the GPU”,
by Hall, Carr and Hart”.
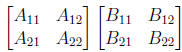
We have been using only the red component, propose storing
across all
colors:
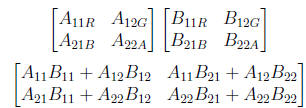
divide into 2:
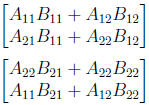
Basically a swizzle operation to
speed things up.
Closing thought: The basic idea here is using the inner product calculation
in parellel.